Next Best Offer (NBO)
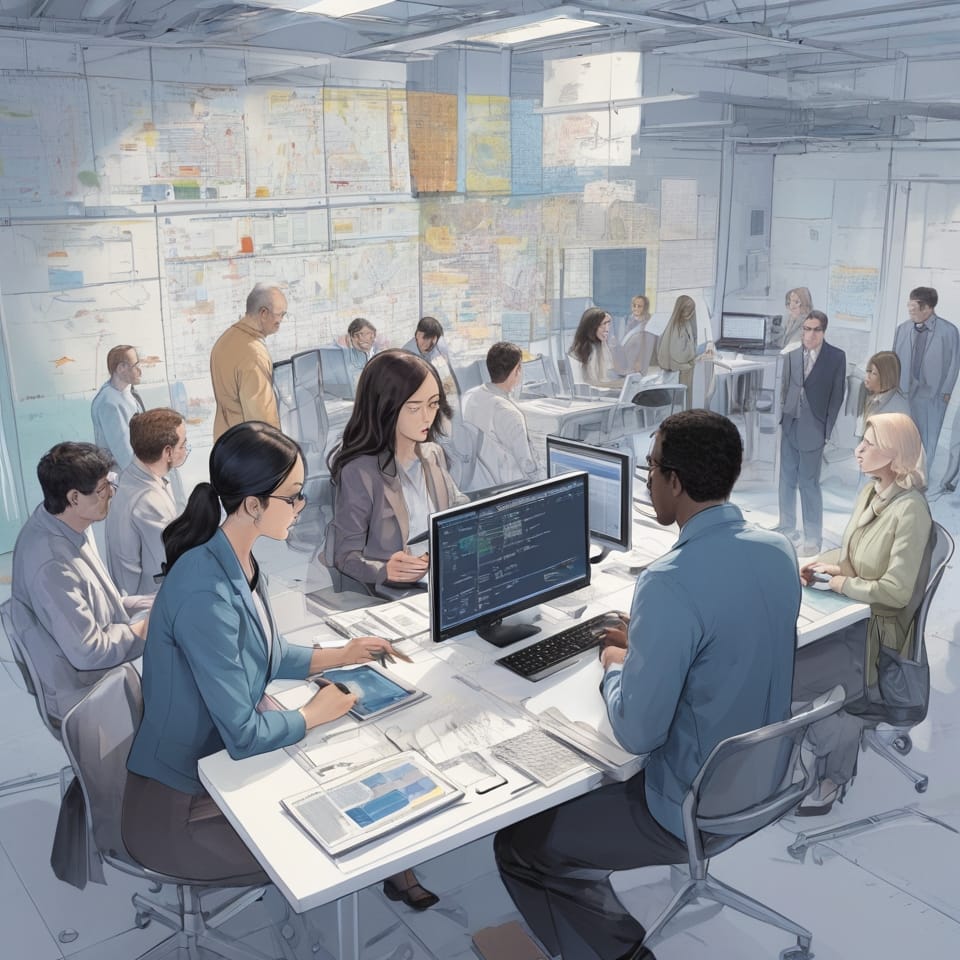
เป็นวิธีการวิเคราะห์เชิงคาดการณ์ที่ใช้เพื่อทราบถึงผลิตภัณฑ์หรือบริการที่เหมาะสมที่สุดที่จะเสนอให้กับลูกค้าในครั้งถัดไป โดยอิงจากคุณลักษณะและพฤติกรรมของลูกค้า
ในบริษัทประกันภัย NBO สามารถช่วยบริษัทแนะนำผลิตภัณฑ์ประกันภัยที่เกี่ยวข้องที่สุดให้กับลูกค้า เพิ่มโอกาสในการขายต่อยอด (Cross selling) รวมถึงสร้างความพึงพอใจให้ลูกค้า มีกระบวนการดังนี้
- การเก็บรวบรวมข้อมูล: รวบรวมข้อมูลลูกค้า รวมถึงข้อมูลประชากร กรมธรรม์ปัจจุบัน ประวัติการเรียกร้องสินไหม และการมี Interaction กับบริษัท
- การสร้าง Features: สร้าง Features ที่เกี่ยวข้องซึ่งสามารถช่วยคาดการณ์ความชอบและความต้องการของลูกค้า
- การ Train model: ใช้ Machine Learning algorithms เพื่อ Train model คาดการณ์ความน่าจะเป็นที่ลูกค้าจะยอมรับข้อเสนอ (Offer) ต่างๆ
- การให้คะแนน (Scoring): ใช้ Model เพื่อให้คะแนนแต่ละข้อเสนอที่เป็นไปได้สำหรับลูกค้าแต่ละราย
- การเสนอ (Offer): เลือกข้อเสนอที่ได้คะแนนสูงสุดเป็น Next Best Offer
ตัวอย่าง Python code
import pandas as pd
import numpy as np
from sklearn.model_selection import train_test_split
from sklearn.preprocessing import StandardScaler
from sklearn.ensemble import RandomForestClassifier
from sklearn.metrics import accuracy_score, classification_report
# ขั้นตอนที่ 1: การเก็บรวบรวมข้อมูล (สมมติว่ามีไฟล์ CSV ที่เป็นข้อมูลลูกค้า)
data = pd.read_csv('insurance_customer_data.csv')
# ขั้นตอนที่ 2: การสร้าง Features
def engineer_features(df):
# ตัวอย่างการสร้าง Features
df['age_group'] = pd.cut(df['age'], bins=[0, 25, 35, 45, 55, 65, 100], labels=['18-25', '26-35', '36-45', '46-55', '56-65', '65+'])
df['policy_duration'] = (pd.Timestamp.now() - pd.to_datetime(df['policy_start_date'])).dt.days / 365
df['claim_ratio'] = df['total_claims'] / df['policy_duration']
return df
data = engineer_features(data)
# เตรียม Features และตัวแปร Target
features = ['age', 'income', 'policy_duration', 'claim_ratio', 'customer_loyalty_score']
X = data[features]
y = data['accepted_offer'] # สมมติว่า Column นี้มีอยู่และบ่งชี้ว่าข้อเสนอก่อนหน้านี้ได้รับการยอมรับหรือไม่
# แบ่งข้อมูล train / test
X_train, X_test, y_train, y_test = train_test_split(X, y, test_size=0.2, random_state=42)
# ทำ Feature scaling
scaler = StandardScaler()
X_train_scaled = scaler.fit_transform(X_train)
X_test_scaled = scaler.transform(X_test)
# ขั้นตอนที่ 3: การ Train model
model = RandomForestClassifier(n_estimators=100, random_state=42)
model.fit(X_train_scaled, y_train)
# การ Evaluate model
y_pred = model.predict(X_test_scaled)
print("Model accuracy:", accuracy_score(y_test, y_pred))
print("\n Classification report:\n", classification_report(y_test, y_pred))
# ขั้นตอนที่ 4: การให้คะแนน (Scoring)
def score_offers(customer_data, offer_list):
customer_features = scaler.transform(customer_data[features].values.reshape(1, -1))
offer_scores = {}
for offer in offer_list:
# สมมติว่าเรามีวิธีในการ Encode ข้อเสนอ ไปสู่ Feature space
offer_features = encode_offer(offer)
combined_features = np.hstack((customer_features, offer_features))
score = model.predict_proba(combined_features)[0][1] # ความน่าจะเป็นที่จะยอมรับข้อเสนอ
offer_scores[offer] = score
return offer_scores
# ขั้นตอนที่ 5: การเสนอ (Offer)
def get_next_best_offer(customer_data, offer_list):
scores = score_offers(customer_data, offer_list)
return max(scores, key=scores.get)
# ตัวอย่างการใช้งาน
customer = data.iloc[0] # ดึงลูกค้าคนแรกเป็นตัวอย่าง
available_offers = ['auto_insurance', 'home_insurance', 'life_insurance', 'travel_insurance']
next_best_offer = get_next_best_offer(customer, available_offers)
print(f"Next Best Offer is: {next_best_offer}")
แสดงตัวอย่าง NBO อย่างง่ายที่ใช้สำหรับบริษัทประกันภัย มีการทำงาน ดังนี้
- Load ข้อมูลลูกค้าและสร้าง Features ที่เกี่ยวข้อง
- เตรียมข้อมูลและแบ่งออกเป็นชุดสำหรับ Train และ Test
- Train model โดยใช้ Random Forest Classifier เพื่อคาดการณ์การยอมรับข้อเสนอ
- สร้าง Function เพื่อให้คะแนนข้อเสนอต่างๆ สำหรับลูกค้าที่กำหนด
- Offer ผลิตภัณฑ์หรือบริการที่ได้คะแนนสูงสุดเป็น Next Best Offer
ในสถานการณ์จริง อาจต้อง
- ใช้เทคนิคการสร้าง Features ที่ซับซ้อนมากขึ้น
- พิจารณาข้อมูลลูกค้าและการมี Interaction ที่หลากหลายมากขึ้น
- อาจใช้ Algorithms ที่มีประสิทธิภาพมากกว่า (เช่น Boosting)
- สร้างระบบเพื่อปรับปรุง Model ด้วยข้อมูลใหม่อย่างต่อเนื่อง
- รวมกฎทางธุรกิจและข้อจำกัดในกระบวนการเลือกข้อเสนอ
- ตรวจสอบและทดสอบ Model อย่างละเอียดก่อนนำไปใช้
Blog นี้ เขียนร่วมกับ Claude.ai โดยใช้ Prompt
As a data scientist in an insurance company, please explain the next best offer model with Python code.