Machine Learning Pipeline
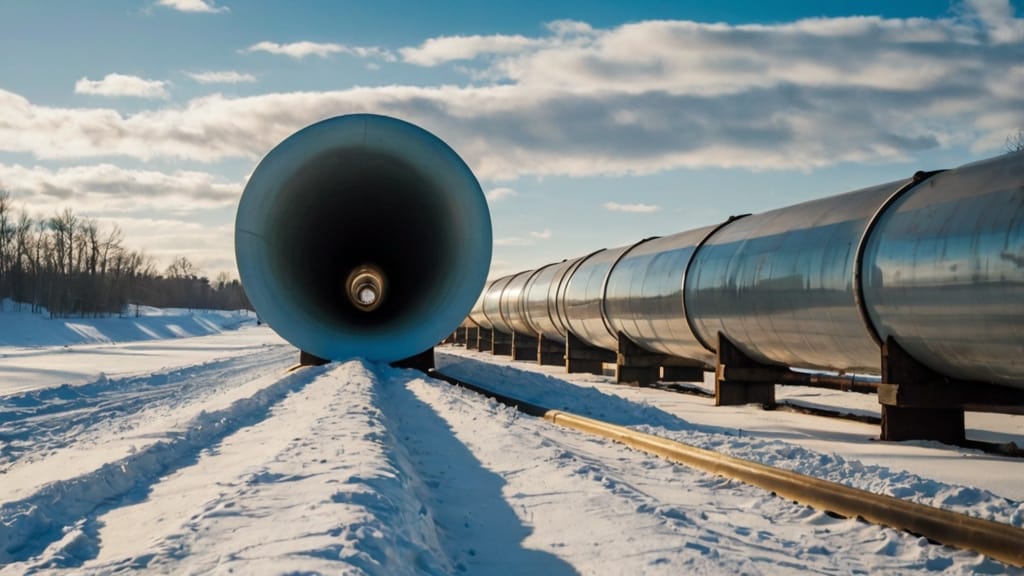
คือ วิธีการแบบอัตโนมัติสำหรับขั้นตอนการทำงานของ Machine Learning Model ตั้งแต่การเตรียมข้อมูลไปจนถึงการประเมิน Model (Evaluation) เป็นลำดับของขั้นตอนต่างๆ ในการประมวลผลข้อมูล โดยผลลัพธ์ของขั้นตอนก่อนหน้า จะกลายเป็นข้อมูล Input ของส่วนถัดไป โดย Pipeline จะช่วยให้
- จัดระเบียบ Code
- ป้องกันการรั่วไหลของข้อมูล
- ทำให้ขั้นตอนการทำงานของ Machine Learning เป็นอัตโนมัติ
- ทำให้ Model สามารถทำซ้ำได้ง่ายขึ้น
Pipeline ทั่วไปของ Machine Learning อาจประกอบด้วยขั้นตอนดังนี้
- การ Load ข้อมูล
- การเตรียมข้อมูล “data preparation” (จัดการค่าที่หายไป, แปลง Category variable ฯลฯ)
- การทำ Feature Scaling
- การทำ Feature Selection
- การ Train model
- การประเมิน Model (Evaluation)
ตัวอย่างแบบง่าย โดยใช้ Library -> Scikit-learn ซึ่งเป็น Python Library ที่นิยม
import numpy as np
from sklearn.datasets import load_iris
from sklearn.model_selection import train_test_split
from sklearn.preprocessing import StandardScaler
from sklearn.impute import SimpleImputer
from sklearn.pipeline import Pipeline
from sklearn.ensemble import RandomForestClassifier
from sklearn.metrics import accuracy_score, classification_report
# Load the iris dataset
iris = load_iris()
X, y = iris.data, iris.target
# Split the data into training and testing sets
X_train, X_test, y_train, y_test = train_test_split(X, y, test_size=0.2, random_state=42)
# Define the pipeline
pipeline = Pipeline([
('imputer', SimpleImputer(strategy='mean')), # Handle missing values
('scaler', StandardScaler()), # Standardize features
('classifier', RandomForestClassifier(n_estimators=100, random_state=42)) # Random Forest classifier
])
# Fit the pipeline on the training data
pipeline.fit(X_train, y_train)
# Make predictions on the test data
y_pred = pipeline.predict(X_test)
# Evaluate the model
accuracy = accuracy_score(y_test, y_pred)
print(f"Accuracy: {accuracy:.2f}")
# Print the classification report
print("\nClassification Report:")
print(classification_report(y_test, y_pred, target_names=iris.target_names))
Pipeline นี้ เป็นการสร้าง Classification Model จากข้อมูล Iris มีองค์ประกอบหลัก ดังนี้
- การ Load และแบ่งข้อมูล: Load ชุดข้อมูล Iris และแบ่งเป็นข้อมูล Train และ Test
- การกำหนด Pipeline: สร้าง Object Pipeline ของ Scikit-learn ในที่นี้ มี 3 ขั้นตอน
- SimpleImputer: จัดการกับค่าที่หายไปโดยแทนที่ด้วยค่าเฉลี่ยของคอลัมน์
- StandardScaler: ปรับค่า Features ต่างๆ ให้เป็นมาตรฐานโดยลบค่าเฉลี่ยออกและปรับ Scale ให้มีความแปรปรวนเท่ากับ 1
- RandomForestClassifier: เลือก Algorithm เป็น Random Forest เพื่อใช้สำหรับ Classification
- การ Train model: ใช้ pipeline ทั้งหมดกับข้อมูล Trainโดยใช้คำสั่ง fit()
- การ Predict และ Evaluate: ใช้ pipeline เพื่อทำนายผลลัพธ์บนข้อมูล Test และประเมินประสิทธิภาพของ Model
ข้อดีของการใช้ pipeline คือ จะรวมทุกขั้นตอนของการสร้าง Machine Learning ไว้ใน Object เดียว ทำให้ง่ายต่อการใช้งาน (ทั้งข้อมูล Train และ Test) ลดโอกาสการรั่วไหลของข้อมูล และทำให้ Code เป็นระเบียบมากขึ้น
✍🏼 Blog นี้ เขียนร่วมกับ Claude.ai โดยใช้ Prompt
Please explain about machine learning pipeline with sample Python code.