Logistic Regression คือ อะไร
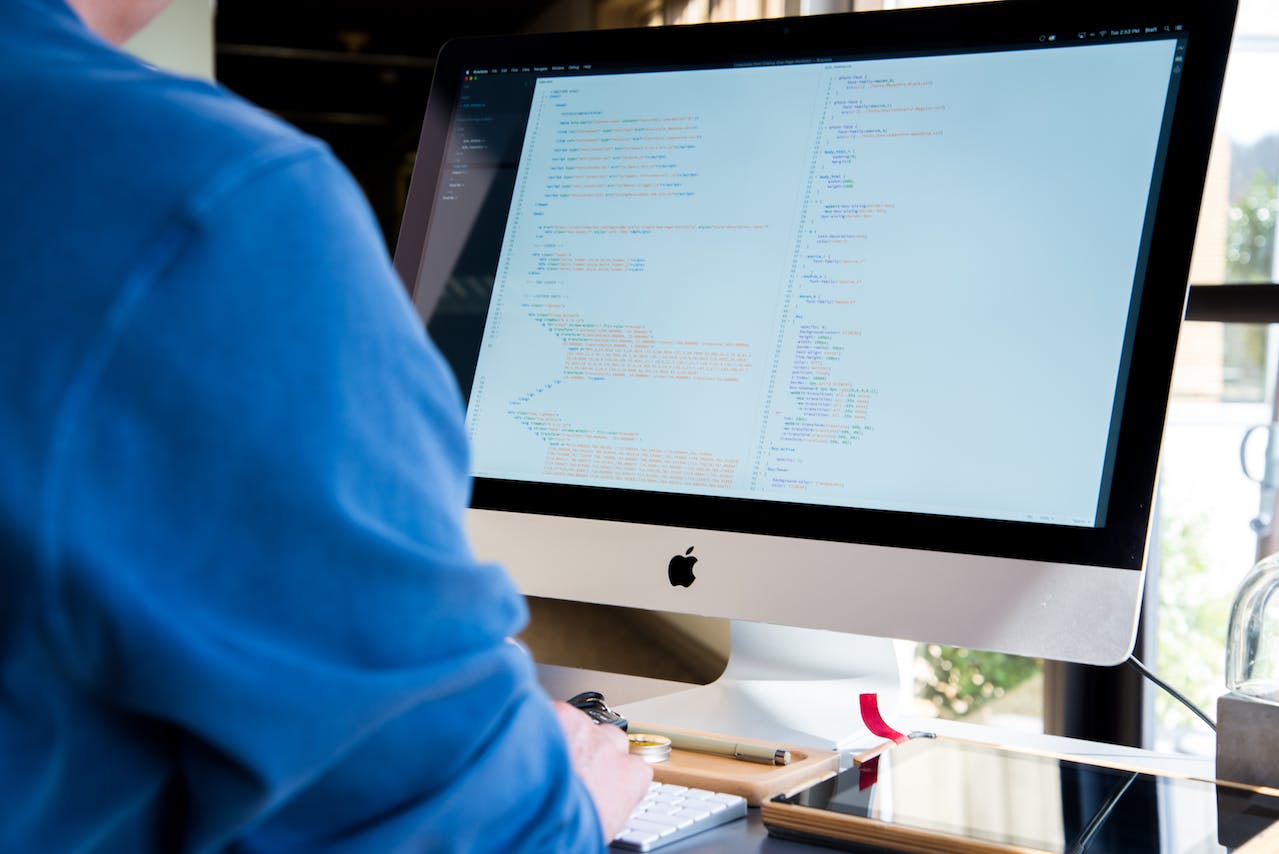
ใช้สำหรับปัญหา Classification ใช้ในการประมาณความน่าจะเป็นที่ Instance จะไปอยู่ใน Class หนึ่งๆ เช่น
- ทำนายนักเรียนที่จะสอบผ่าน หรือ ไม่ผ่าน จากจำนวนชั่วโมงเข้าเรียน
- ทำนาย Transaction ว่าเป็น Fraud หรือไม่
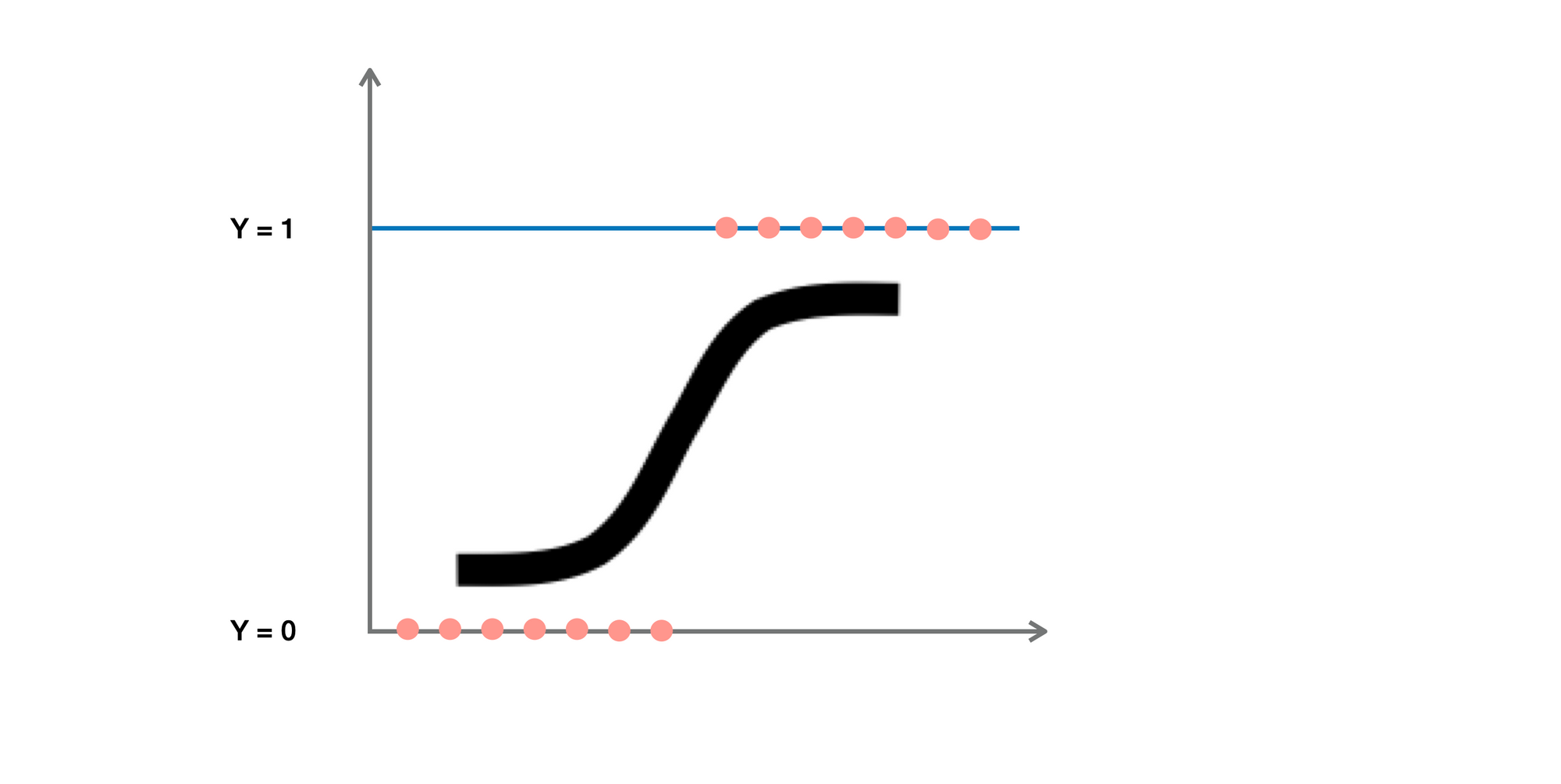
🟢 ข้อดี
- แน้วโน้มที่จะเกิด Overfit น้อย แต่เป็นไปได้ กรณี High Dimensional Datasets
- ใช้งานได้ดี เมื่อ Dataset มีความสัมพันธ์แบบ Linear
- ง่ายในการ Implement และ ใช้เวลาในการ Train น้อย
- แปลความหมายได้ง่าย
🔴 ข้อเสีย
- จากสมมติฐาน ต้องเป็น Linear Boundary
- ไม่มีความสามารถในการทำงานกับความสัมพันธ์ที่ซับซ้อนมากได้
Hyper-parameters ใน Logistic Regression
- C (Inverse ของ regularization strength)
- penalty (ถูกใช้ในการระบุ Norm ใน Penalization)
- solver (Algorithm ที่ใช้สำหรับ Optimization)
- max_iter (จำนวน Iterations สูงสุด สำหรับ Solvers ที่จะ Converge)
- fit_intercept (ระบุหากมี Constant ที่ต้องเพิ่มใน Decision Function)
- multi_class (กรณีใช้กับปัญหา Multi-classes)
ตัวอย่าง Python Code 👨🏻💻
# To import libraries
import numpy as np
from sklearn.linear_model import LogisticRegression
from sklearn.datasets import load_iris
from sklearn.model_selection import train_test_split, GridSearchCV
from sklearn.metrics import classification_report
# To load dataset
data = load_iris()
X = data.data
y = data.target
# To split train and test data
X_train, X_test, y_train, y_test = train_test_split(X, y, test_size=0.25, random_state=0)
# Initialize Logistic Regression
clf = LogisticRegression()
# To define hyper-parameters grid for tuning
param_grid = {
'C': np.logspace(-4, 4, 20),
'penalty':['l2','none'],
'solver':['newton-cg','lbfs','liblinear','sag','saga'],
'max_iter':[100, 500, 1000, 1500]
}
# To use GridSerachCV to find the best hyperparameters
grid_search = GridSearchCV(clf, param_grid=param_grid, cv=5, verbose=1, n_jobs=-1)
grid_search.fit(X_train, y_train)
# To print the best hyper-parameters
print ("Best Parameters: ", grid_search.best_params_)
# To use the best model for predicting the test data
best_clf = grid_search.best_estimator_
predictions = best_clf.predict(X_test)
# To print the classification report
print (classification_report(y_test, predictions))
******
อ่านเพิ่มเติม Pros & Cons ของ Machine Learning Algorithms ที่นิยมใช้
******
ข้อมูลอ้างอิง - Analytics Vidhya