K-Fold Cross Validation
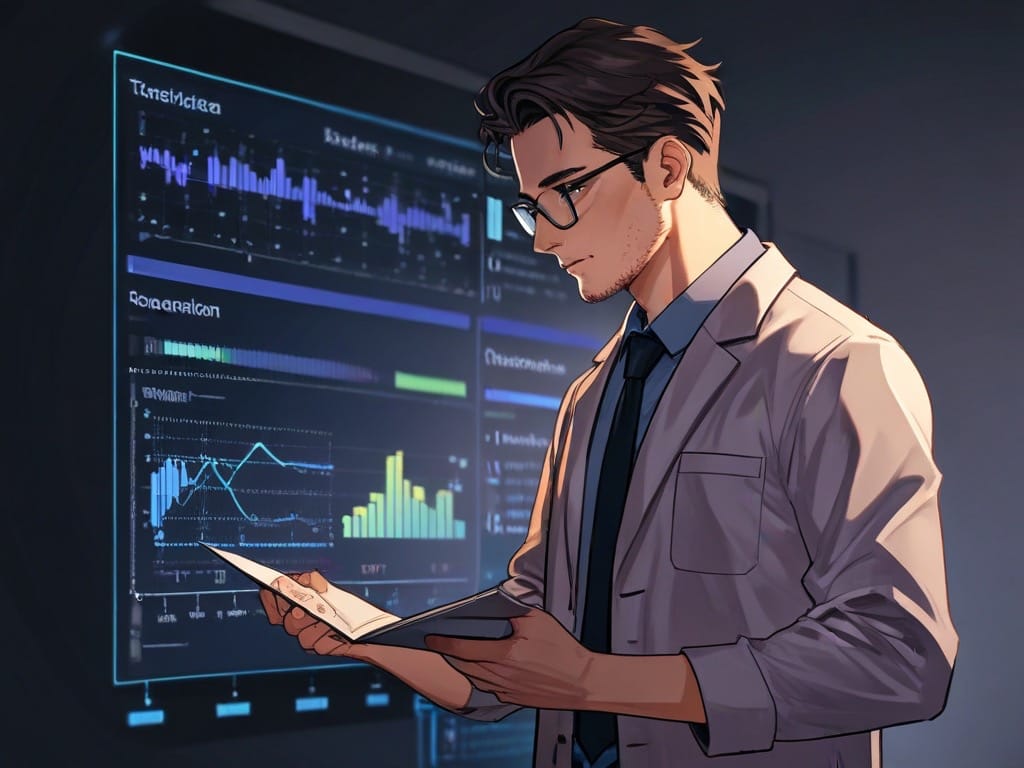
K-fold Cross Validation เป็นเทคนิคที่มีประสิทธิภาพถูกนำมาใช้ใน Machine Learning (ML) เพื่อประเมิน (Evaluate) ประสิทธิภาพของ Model ป้องกันการเกิด Overfitting โดยทำการ Split dataset ไปเป็น k subsets ทำการ Train model จำนวน k ครั้ง ที่ Subsets ต่างๆ กัน จากนั้น ทำการหาค่าเฉลี่ยของประสิทธิภาพ Model เช่น ค่า Accuracy กระบวนการนี้ จะช่วยให้ประเมินได้ว่า Model สามารถทำงานกับ Unseen data ได้ดีเพียงใด
สามารถใช้ Python ได้ โดยมีขั้นตอนการทำงาน ดังนี้
1) เรียกใช้งาน Libraries
from sklearn.model_selection import KFold
from sklearn.linear_model import LogisticRegression
from sklearn.metrics import accuracy_score
import numpy as np
2) เตรียมข้อมูล (Data Preparation)
ในที่นี้ ให้ X เป็น Feature matrix และ y เป็น Target variable
3) สร้าง K-Fold object
โดยทำการแบ่งเป็น 5 Folds (n_splits=5) เลือก shuffle=True เพื่อให้แน่ใจว่า Data มีการ Shuffle ก่อนที่จะ Splitting และ กำหนด random_state=42 เป็นจุดเริ่มต้นในการ Seed เพื่อประโยชน์ในการทดลองซ้ำๆ
kf = KFold(n_splits=5, shuffle=True, random_state=42)
4) ทำ K-Fold Cross Validation
ซึ่งจะเป็นการทำซ้ำๆ แต่ละ Fold โดยใช้ kf.split(X)
- ทำการ Split data ไปเป็น Training และ Test sets โดยใช้ kf.split(X)
- ทำการ Train logistic regression model ที่ Training set
- ทำการ Predict ที่ Test set โดยใช้ Model ที่ Train มา
- ทำการคำนวณค่า Accuracy ในแต่ละ Fold โดยใช้ accuracy_score.
- ทำการ Append ค่า Accuracy score ไปที่ตัวแปร scores
scores = []
for train_index, test_index in kf.split(X):
X_train, X_test = X[train_index], X[test_index]
y_train, y_test = y[train_index], y[test_index]
# Train the model
model = LogisticRegression()
model.fit(X_train, y_train)
# Evaluate the model
y_pred = model.predict(X_test)
score = accuracy_score(y_test, y_pred)
scores.append(score)
print(f"Accuracy scores for each fold: {scores}")
print(f"Average accuracy: {np.mean(scores)}")
แสดงผล Accuracy score สำหรับแต่ละ Fold และ ค่าเฉลี่ย Accuracy จากทุก Folds ผลลัพธ์ที่ได้ ดังนี้
Accuracy scores for each fold: [0.82, 0.79, 0.85, 0.81, 0.78]
Average accuracy: 0.81
ในตัวอย่างนี้ ใช้ Logistic regression แต่สามารถแทนที่ด้วย Algorithms ใดๆ ก็ได้ ขั้นตอนสำคัญ คือ การสร้าง KFold object และ การ Evaluate ซ้ำๆ (Iteration) ในแต่ละ Fold และคำนวณ Performance metric (ในตัวอย่าง เลือกใช้ Accuracy)
K-fold Cross Validation ช่วยในการประเมินประสิทธิภาพของ Model และน่าเชื่อถือกว่า การใช้ Train-test split เพียงครั้งเดียว การทำ Split หลายๆ ครั้ง แล้วพิจารณาค่าเฉลี่ยของ Performance metric ทำให้ประเมินได้ว่า เมื่อ Model ทำงานกับ Unseen data จะให้ผลลัพธ์อย่างไร
หมายเหตุ: Blog นี้ เขียนร่วมกับ Claude.ai โดยใช้ Prompt ดังนี้
As a nerd data scientist, please explain k-folds cross validation with python code.